Bloomberg’s 6 Notable Academic Contributions in Machine Learning in 2016
March 17, 2017
Machine learning, especially natural language processing (NLP), has become a hot topic in the technology world. It’s also a discipline that’s been gaining a growing amount of attention at Bloomberg, where more than 100 technologists and data scientists are devoted to machine learning and NLP applications. Bloomberg’s engineers have been hard at work throughout the past year making advances in the area, publishing papers, teaching college courses, attending conferences and developing projects that showcase their expertise in this space. Their work has also helped improve the company’s products and services, giving its customers a significant competitive edge.
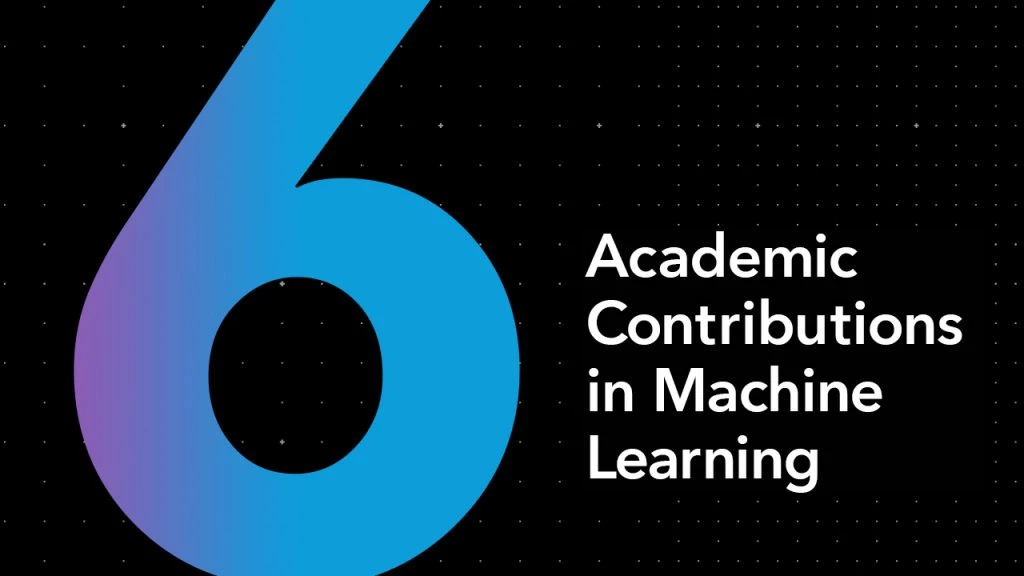
Here’s a summary of six of the notable contributions by various machine learning experts at Bloomberg in the academic realm in 2016:
Ranking Financial Tweets
Financial markets move with the news, no matter where it’s reported. This includes Twitter, which has become a highly regarded news source that complements traditional outlets. While financial traders can read tweets on the Bloomberg Terminal, they can’t always tell whether they are novel, relevant, rumor or speculation. This paper, which was published at SIGIR 2016 in July 2016, was written by senior NLP researcher Miles Osborne, together with two engineers on Bloomberg’s News Search Experience team in London, Diego Ceccarelli and Francesco Nidito. It examines the myriad of factors that help determine which tweets are relevant for investment decisions. In the future, our engineers will be able to incorporate such innovations into the design of the next generation of machine-learning powered news rankers, ensuring our algorithms will be able to better predict which tweets customers will need, and want, to read.
Utilizing Knowledge Bases in Text-centric Information Retrieval
Knowledge graphs are database-like structures that facilitate the retrieval of related information. They allow computers to consume (and reason about) this information, enabling smarter applications through a form of artificial intelligence. The tutorial workshop was originally presented as a tutorial at ICTIR 2016 last September by Edgar Meij, a senior data scientist on our News Search Experience team in London, together with Laura Dietz of the University of New Hampshire and Alexander Kotov of Wayne State University. It helped disseminate best practices, as well as recent advances in both academic literature and industry regarding the use of knowledge graphs to improve information retrieval. The group delivered this tutorial again last month at WSDM 2017 (view the slides).
User Intent in Online Video Search
Last September, Dr. Christoph Kofler, a software engineer and data scientist on the Unified Search Application/Data Science team, had his Ph.D. dissertation about deciphering user intent in multimedia search engines recognized as the 2016 ACM Special Interest Group on Multimedia (SIGMM) Outstanding Ph.D. Thesis Award. In November, he published a related survey of multimedia information retrieval research directed at the problem of enabling search engines to respond to user intent in ACM Computing Surveys (CSUR), in collaboration with Delft University of Technology’s Martha Larson and Alan Hanjalic.
Temporal Causal Modeling
Traders, portfolio managers and other financial market participants need to stay ahead of fast-moving markets. One approach is to observe correlations between different companies and changes in their stock prices, or how tweets impact stock prices. Senior NLP researcher Prabhanjan (Anju) Kambadur co-authored a chapter in “Financial Signal Processing and Machine Learning,” a book published by Wiley in May 2016. In it, he explores another potential perspective – that there are causal relationships in multivariate time series data – like stock prices. By understanding and mapping these causal relationships among assets, portfolio managers may be able to better understand and predict market behaviors and then develop a strategy that mitigates their investment risk accordingly.
Mention2Vec: Entity Identification as Multitasking
Customers rely on Bloomberg for information and data they can access quickly and reliably. Most importantly, they need it to be accurate, which currently requires significant manual effort on our part. With a greater focus on machine learning and automation, we’re starting to depend less on human input and more on the power of named-entity recognition (NER) as a first step in information extraction. This new NER model, which is outlined in senior NLP researcher Karl Stratos’ paper that was posted to arXiv in December 2016, is ten times faster in scanning and grabbing financial data from corporate filings, for example – with much greater accuracy.
Machine Learning and Computational Statistics
As part of the Data Science team in the office of the CTO, David Rosenberg helps set the technical direction for machine learning projects throughout the Engineering and Global Data departments. When he’s not working to keep Bloomberg’s technology ahead of the competition, Rosenberg is an adjunct associate professor at New York University’s Center for Data Science (CDS), teaching DS-GA 1003. This graduate-level course covers a wide variety of topics in machine learning and statistical modeling, and serves as part of the core curriculum towards earning a Master’s degree in Data Science. Students appreciate Rosenberg as much as we do, voting him CDS Professor of the Year in both 2015 and 2016 – a well-deserved accolade from the next generation of data scientists.
More to come in 2017
Engineers at Bloomberg are already hard at work on exciting machine learning projects and other academic contributions. In addition to teaching at leading universities throughout New York City and helping organize prestigious industry conferences, a number of them plan to continue publishing papers that will further advance machine learning, for both Bloomberg’s customers and the industry as a whole. We look forward to sharing more about these efforts throughout the year.